Predicting Sentiments of FOMC Corpus#
Analyse statement by Loughran and McDonald dictionary, finbert, and T5 model
%config InlineBackend.figure_format='retina'
from ekorpkit import eKonf
logging.basicConfig(level=logging.WARNING)
print("version:", eKonf.__version__)
print("is notebook?", eKonf.is_notebook())
print("is colab?", eKonf.is_colab())
print("evironment varialbles:")
eKonf.print(eKonf.env().dict())
version: 0.1.33+28.g90d1dea
is notebook? True
is colab? False
evironment varialbles:
{'EKORPKIT_CONFIG_DIR': '/workspace/projects/ekorpkit-book/config',
'EKORPKIT_DATA_DIR': None,
'EKORPKIT_PROJECT': 'ekorpkit-book',
'EKORPKIT_WORKSPACE_ROOT': '/workspace',
'NUM_WORKERS': 230}
start_year = 2000
data_dir = "../data/fomc"
eKonf.env().FRED_API_KEY
pydantic.types.SecretStr
Predict sentiments with the LM sentiment analyser#
Load FOMC Corpus#
fomc_sents = eKonf.load_data("fomc_sents.parquet", data_dir)
fomc_sents.tail()
id | text | split | timestamp | content_type | date | speaker | title | decision | rate | recent_meeting | recent_decision | recent_rate | next_meeting | next_decision | next_rate | text_num_words | section_id | sent_id | |
---|---|---|---|---|---|---|---|---|---|---|---|---|---|---|---|---|---|---|---|
653463 | 2854 | It will not have the word “somewhat” on line 3. | train | 2014-12-17 | fomc_meeting_script | 2014-12-17 | MR. LUECKE | FOMC Meeting Transcript | 0.0 | 0.25 | 2014-12-17 | 0.0 | 0.25 | 2015-01-28 | 0.0 | 0.25 | 10 | 287 | 2 |
653464 | 2854 | Chair Yellen Yes Vice Chairman Dudley ... | train | 2014-12-17 | fomc_meeting_script | 2014-12-17 | MR. LUECKE | FOMC Meeting Transcript | 0.0 | 0.25 | 2014-12-17 | 0.0 | 0.25 | 2015-01-28 | 0.0 | 0.25 | 31 | 287 | 4 |
653465 | 2854 | And let me confirm that the next meeting will ... | train | 2014-12-17 | fomc_meeting_script | 2014-12-17 | CHAIR YELLEN | FOMC Meeting Transcript | 0.0 | 0.25 | 2014-12-17 | 0.0 | 0.25 | 2015-01-28 | 0.0 | 0.25 | 19 | 288 | 3 |
653466 | 2854 | I believe box lunches are now available for pe... | train | 2014-12-17 | fomc_meeting_script | 2014-12-17 | CHAIR YELLEN | FOMC Meeting Transcript | 0.0 | 0.25 | 2014-12-17 | 0.0 | 0.25 | 2015-01-28 | 0.0 | 0.25 | 33 | 288 | 4 |
653467 | 2854 | I will do my best, and I will consider at the ... | train | 2014-12-17 | fomc_meeting_script | 2014-12-17 | CHAIR YELLEN | FOMC Meeting Transcript | 0.0 | 0.25 | 2014-12-17 | 0.0 | 0.25 | 2015-01-28 | 0.0 | 0.25 | 18 | 288 | 5 |
Predict sentiments of sentences#
model_cfg = eKonf.compose('model/sentiment=lm')
model_cfg.num_workers = 100
lmsa = eKonf.instantiate(model_cfg)
article = fomc_sents.text[10]
lmsa.predict_sentence(article)
{'num_tokens': 156,
'polarity': -0.9999990000010001,
'polarity_label': 'negative',
'uncertainty': 1e-06}
fomc_sent_sentiments = lmsa.predict(fomc_sents)
eKonf.save_data(fomc_sent_sentiments, "fomc_sent_sentiments.parquet", data_dir)
INFO:ekorpkit.models.sentiment.lbsa:Predicting sentiments of the column [text] using predict_sentence
INFO:ekorpkit.base:Using batcher with minibatch size: 1000
INFO:ekorpkit.utils.batch.batcher: backend: joblib minibatch_size: 1000 procs: 100 input_split: False merge_output: True len(data): 653468 len(args): 5
Predicting [text]: 100%|██████████| 654/654 [02:50<00:00, 3.83it/s]
INFO:ekorpkit.models.sentiment.lbsa: >> elapsed time to predict: 0:02:51.902053
id | text | split | timestamp | content_type | date | speaker | title | decision | rate | ... | next_meeting | next_decision | next_rate | text_num_words | section_id | sent_id | num_tokens | polarity | polarity_label | uncertainty | |
---|---|---|---|---|---|---|---|---|---|---|---|---|---|---|---|---|---|---|---|---|---|
0 | 0 | The Secretary reported that advices of the ele... | train | 1993-02-03 | fomc_minutes | 1993-02-03 | Alan Greenspan | FOMC Meeting Minutes | 0.0 | 3.00 | ... | 1993-02-18 | 0.0 | 3.00 | 47 | 29 | 0 | 52 | 0.000000 | neutral | 0.000001 |
1 | 0 | By unanimous vote, the Committee elected the f... | train | 1993-02-03 | fomc_minutes | 1993-02-03 | Alan Greenspan | FOMC Meeting Minutes | 0.0 | 3.00 | ... | 1993-02-18 | 0.0 | 3.00 | 73 | 35 | 0 | 78 | -1.000000 | negative | 0.000001 |
2 | 0 | By unanimous vote, William J. McDonough, Marga... | train | 1993-02-03 | fomc_minutes | 1993-02-03 | Alan Greenspan | FOMC Meeting Minutes | 0.0 | 3.00 | ... | 1993-02-18 | 0.0 | 3.00 | 74 | 37 | 0 | 83 | 1.000000 | positive | 0.000001 |
3 | 0 | On January 15, 1993, the continuing rules, reg... | train | 1993-02-03 | fomc_minutes | 1993-02-03 | Alan Greenspan | FOMC Meeting Minutes | 0.0 | 3.00 | ... | 1993-02-18 | 0.0 | 3.00 | 59 | 39 | 0 | 68 | -0.333333 | negative | 0.014707 |
4 | 0 | Members were asked to indicate if they wished ... | train | 1993-02-03 | fomc_minutes | 1993-02-03 | Alan Greenspan | FOMC Meeting Minutes | 0.0 | 3.00 | ... | 1993-02-18 | 0.0 | 3.00 | 25 | 39 | 1 | 26 | -0.999999 | negative | 0.000001 |
... | ... | ... | ... | ... | ... | ... | ... | ... | ... | ... | ... | ... | ... | ... | ... | ... | ... | ... | ... | ... | ... |
653463 | 2854 | It will not have the word “somewhat” on line 3. | train | 2014-12-17 | fomc_meeting_script | 2014-12-17 | MR. LUECKE | FOMC Meeting Transcript | 0.0 | 0.25 | ... | 2015-01-28 | 0.0 | 0.25 | 10 | 287 | 2 | 13 | 0.000000 | neutral | 0.076924 |
653464 | 2854 | Chair Yellen Yes Vice Chairman Dudley ... | train | 2014-12-17 | fomc_meeting_script | 2014-12-17 | MR. LUECKE | FOMC Meeting Transcript | 0.0 | 0.25 | ... | 2015-01-28 | 0.0 | 0.25 | 31 | 287 | 4 | 31 | 0.000000 | neutral | 0.000001 |
653465 | 2854 | And let me confirm that the next meeting will ... | train | 2014-12-17 | fomc_meeting_script | 2014-12-17 | CHAIR YELLEN | FOMC Meeting Transcript | 0.0 | 0.25 | ... | 2015-01-28 | 0.0 | 0.25 | 19 | 288 | 3 | 21 | 0.000000 | neutral | 0.000001 |
653466 | 2854 | I believe box lunches are now available for pe... | train | 2014-12-17 | fomc_meeting_script | 2014-12-17 | CHAIR YELLEN | FOMC Meeting Transcript | 0.0 | 0.25 | ... | 2015-01-28 | 0.0 | 0.25 | 33 | 288 | 4 | 39 | 0.000000 | neutral | 0.025642 |
653467 | 2854 | I will do my best, and I will consider at the ... | train | 2014-12-17 | fomc_meeting_script | 2014-12-17 | CHAIR YELLEN | FOMC Meeting Transcript | 0.0 | 0.25 | ... | 2015-01-28 | 0.0 | 0.25 | 18 | 288 | 5 | 22 | 0.000000 | neutral | 0.000001 |
653468 rows × 23 columns
fomc_sent_sentiments = eKonf.load_data("fomc_sent_sentiments.parquet", data_dir)
fomc_sent_sentiments
id | text | split | timestamp | content_type | date | speaker | title | decision | rate | ... | next_meeting | next_decision | next_rate | text_num_words | section_id | sent_id | num_tokens | polarity | polarity_label | uncertainty | |
---|---|---|---|---|---|---|---|---|---|---|---|---|---|---|---|---|---|---|---|---|---|
0 | 0 | The Secretary reported that advices of the ele... | train | 1993-02-03 | fomc_minutes | 1993-02-03 | Alan Greenspan | FOMC Meeting Minutes | 0.0 | 3.00 | ... | 1993-02-18 | 0.0 | 3.00 | 47 | 29 | 0 | 52 | 0.000000 | neutral | 0.000001 |
1 | 0 | By unanimous vote, the Committee elected the f... | train | 1993-02-03 | fomc_minutes | 1993-02-03 | Alan Greenspan | FOMC Meeting Minutes | 0.0 | 3.00 | ... | 1993-02-18 | 0.0 | 3.00 | 73 | 35 | 0 | 78 | -1.000000 | negative | 0.000001 |
2 | 0 | By unanimous vote, William J. McDonough, Marga... | train | 1993-02-03 | fomc_minutes | 1993-02-03 | Alan Greenspan | FOMC Meeting Minutes | 0.0 | 3.00 | ... | 1993-02-18 | 0.0 | 3.00 | 74 | 37 | 0 | 83 | 1.000000 | positive | 0.000001 |
3 | 0 | On January 15, 1993, the continuing rules, reg... | train | 1993-02-03 | fomc_minutes | 1993-02-03 | Alan Greenspan | FOMC Meeting Minutes | 0.0 | 3.00 | ... | 1993-02-18 | 0.0 | 3.00 | 59 | 39 | 0 | 68 | -0.333333 | negative | 0.014707 |
4 | 0 | Members were asked to indicate if they wished ... | train | 1993-02-03 | fomc_minutes | 1993-02-03 | Alan Greenspan | FOMC Meeting Minutes | 0.0 | 3.00 | ... | 1993-02-18 | 0.0 | 3.00 | 25 | 39 | 1 | 26 | -0.999999 | negative | 0.000001 |
... | ... | ... | ... | ... | ... | ... | ... | ... | ... | ... | ... | ... | ... | ... | ... | ... | ... | ... | ... | ... | ... |
653463 | 2854 | It will not have the word “somewhat” on line 3. | train | 2014-12-17 | fomc_meeting_script | 2014-12-17 | MR. LUECKE | FOMC Meeting Transcript | 0.0 | 0.25 | ... | 2015-01-28 | 0.0 | 0.25 | 10 | 287 | 2 | 13 | 0.000000 | neutral | 0.076924 |
653464 | 2854 | Chair Yellen Yes Vice Chairman Dudley ... | train | 2014-12-17 | fomc_meeting_script | 2014-12-17 | MR. LUECKE | FOMC Meeting Transcript | 0.0 | 0.25 | ... | 2015-01-28 | 0.0 | 0.25 | 31 | 287 | 4 | 31 | 0.000000 | neutral | 0.000001 |
653465 | 2854 | And let me confirm that the next meeting will ... | train | 2014-12-17 | fomc_meeting_script | 2014-12-17 | CHAIR YELLEN | FOMC Meeting Transcript | 0.0 | 0.25 | ... | 2015-01-28 | 0.0 | 0.25 | 19 | 288 | 3 | 21 | 0.000000 | neutral | 0.000001 |
653466 | 2854 | I believe box lunches are now available for pe... | train | 2014-12-17 | fomc_meeting_script | 2014-12-17 | CHAIR YELLEN | FOMC Meeting Transcript | 0.0 | 0.25 | ... | 2015-01-28 | 0.0 | 0.25 | 33 | 288 | 4 | 39 | 0.000000 | neutral | 0.025642 |
653467 | 2854 | I will do my best, and I will consider at the ... | train | 2014-12-17 | fomc_meeting_script | 2014-12-17 | CHAIR YELLEN | FOMC Meeting Transcript | 0.0 | 0.25 | ... | 2015-01-28 | 0.0 | 0.25 | 18 | 288 | 5 | 22 | 0.000000 | neutral | 0.000001 |
653468 rows × 23 columns
Aggregate sentiment scores#
fomc_tones_lm = lmsa.aggregate_scores(fomc_sent_sentiments, groupby=['content_type', 'date'])
fomc_tones_lm.content_type = fomc_tones_lm.content_type.str.replace('fomc_', '')
eKonf.save_data(fomc_tones_lm, 'fomc_tones_lm.parquet', data_dir)
fomc_tones_lm = eKonf.load_data('fomc_tones_lm.parquet', data_dir)
fomc_tones_lm
content_type | date | polarity_mean | polarity_diffusion | positive | negative | num_tokens_sum | num_tokens_mean | num_tokens_median | num_examples | polarity_mean_label | polarity_diffusion_label | |
---|---|---|---|---|---|---|---|---|---|---|---|---|
0 | beigebook | 2021-01-13 | -0.068247 | -0.071053 | 3972 | 5229 | 397855 | 22.489119 | 21.0 | 17691 | neutral | neutral |
1 | beigebook | 2021-03-03 | -0.032683 | -0.037384 | 3887 | 4518 | 381300 | 22.590201 | 21.0 | 16879 | neutral | neutral |
2 | beigebook | 2021-04-14 | -0.030535 | -0.035085 | 3568 | 4100 | 340121 | 22.430983 | 21.0 | 15163 | neutral | neutral |
3 | beigebook | 2021-06-02 | -0.039873 | -0.044760 | 3837 | 4561 | 357745 | 22.117156 | 21.0 | 16175 | neutral | neutral |
4 | beigebook | 2021-07-14 | 0.011743 | 0.012195 | 191 | 182 | 15803 | 21.413279 | 21.0 | 738 | neutral | neutral |
... | ... | ... | ... | ... | ... | ... | ... | ... | ... | ... | ... | ... |
2390 | testimony | 2021-05-19 | -0.171642 | -0.164179 | 15 | 26 | 2590 | 38.656716 | 29.0 | 67 | negative | negative |
2391 | testimony | 2021-06-22 | 0.220126 | 0.207547 | 20 | 9 | 1456 | 27.471698 | 24.0 | 53 | positive | positive |
2392 | testimony | 2021-07-14 | 0.259259 | 0.305556 | 14 | 3 | 1066 | 29.611111 | 27.0 | 36 | positive | positive |
2393 | testimony | 2021-09-28 | 0.031746 | 0.000000 | 9 | 9 | 1026 | 24.428571 | 22.0 | 42 | neutral | neutral |
2394 | testimony | 2021-11-30 | -0.120000 | -0.200000 | 3 | 7 | 556 | 27.800000 | 26.0 | 20 | neutral | negative |
2395 rows × 12 columns
cfg = eKonf.compose('pipeline/pivot')
cfg.index = 'date'
cfg.columns = 'content_type'
cfg.values = ['polarity_mean', 'polarity_diffusion', 'num_examples', 'num_tokens_sum', 'num_tokens_mean']
tone_data_lm = eKonf.pipe(fomc_tones_lm, cfg)
tone_data_lm = eKonf.to_datetime(tone_data_lm, _columns='date')
tone_data_lm = tone_data_lm.set_index('date')
eKonf.save_data(tone_data_lm, 'fomc_tone_data_lm.parquet', data_dir)
tone_data_lm = eKonf.load_data('fomc_tone_data_lm.parquet', data_dir)
tone_data_lm
polarity_mean_beigebook | polarity_mean_meeting_script | polarity_mean_minutes | polarity_mean_press_conf | polarity_mean_speech | polarity_mean_statement | polarity_mean_testimony | polarity_diffusion_beigebook | polarity_diffusion_meeting_script | polarity_diffusion_minutes | ... | num_tokens_sum_speech | num_tokens_sum_statement | num_tokens_sum_testimony | num_tokens_mean_beigebook | num_tokens_mean_meeting_script | num_tokens_mean_minutes | num_tokens_mean_press_conf | num_tokens_mean_speech | num_tokens_mean_statement | num_tokens_mean_testimony | |
---|---|---|---|---|---|---|---|---|---|---|---|---|---|---|---|---|---|---|---|---|---|
date | |||||||||||||||||||||
1990-02-07 | NaN | -0.087583 | NaN | NaN | NaN | NaN | NaN | NaN | -0.095663 | NaN | ... | NaN | NaN | NaN | NaN | 30.213010 | NaN | NaN | NaN | NaN | NaN |
1990-03-27 | NaN | -0.171992 | NaN | NaN | NaN | NaN | NaN | NaN | -0.179702 | NaN | ... | NaN | NaN | NaN | NaN | 29.846369 | NaN | NaN | NaN | NaN | NaN |
1990-05-15 | NaN | -0.116052 | NaN | NaN | NaN | NaN | NaN | NaN | -0.125461 | NaN | ... | NaN | NaN | NaN | NaN | 29.749077 | NaN | NaN | NaN | NaN | NaN |
1990-07-03 | NaN | -0.114829 | NaN | NaN | NaN | NaN | NaN | NaN | -0.117794 | NaN | ... | NaN | NaN | NaN | NaN | 29.667920 | NaN | NaN | NaN | NaN | NaN |
1990-08-21 | NaN | -0.209552 | NaN | NaN | NaN | NaN | NaN | NaN | -0.219403 | NaN | ... | NaN | NaN | NaN | NaN | 31.032836 | NaN | NaN | NaN | NaN | NaN |
... | ... | ... | ... | ... | ... | ... | ... | ... | ... | ... | ... | ... | ... | ... | ... | ... | ... | ... | ... | ... | ... |
2021-11-30 | NaN | NaN | NaN | NaN | -0.167014 | NaN | -0.12 | NaN | NaN | NaN | ... | 3066.0 | NaN | 556.0 | NaN | NaN | NaN | NaN | 31.937500 | NaN | 27.8 |
2021-12-01 | -0.046022 | NaN | NaN | NaN | NaN | NaN | NaN | -0.048109 | NaN | NaN | ... | NaN | NaN | NaN | 22.539497 | NaN | NaN | NaN | NaN | NaN | NaN |
2021-12-02 | NaN | NaN | NaN | NaN | -0.077381 | NaN | NaN | NaN | NaN | NaN | ... | 6514.0 | NaN | NaN | NaN | NaN | NaN | NaN | 36.188889 | NaN | NaN |
2021-12-15 | NaN | NaN | -0.043929 | -0.075441 | NaN | 0.166667 | NaN | NaN | NaN | -0.064286 | ... | NaN | 489.0 | NaN | NaN | NaN | 30.521429 | 37.587413 | NaN | 27.166667 | NaN |
2021-12-17 | NaN | NaN | NaN | NaN | -0.356613 | NaN | NaN | NaN | NaN | NaN | ... | 3694.0 | NaN | NaN | NaN | NaN | NaN | NaN | 29.317460 | NaN | NaN |
1876 rows × 35 columns
Predict sentiments and aggregate scores with a pipeline#
model_cfg = eKonf.compose('model/sentiment=lm')
cfg = eKonf.compose('pipeline')
cfg._pipeline_ = ['predict', 'aggregate_scores', 'replace', 'pivot', 'save_dataframe']
cfg.num_workers = 100
cfg.data.data_file = "fomc_sents.parquet"
cfg.data.data_dir = data_dir
cfg.predict.model = model_cfg
cfg.predict.path.output.base_dir = data_dir
cfg.predict.path.output.filename = "fomc_sent_sentiments.parquet"
cfg.aggregate_scores.groupby = ['content_type', 'date']
cfg.replace.apply_to = 'content_type'
cfg.replace.rcParams.to_replace = {'fomc_': ''}
cfg.replace.rcParams.regex = True
cfg.pivot.index = 'date'
cfg.pivot.columns = 'content_type'
cfg.pivot.values = ['polarity_mean', 'polarity_diffusion', 'num_examples', 'num_tokens_sum', 'num_tokens_mean']
cfg.save_dataframe.output_dir = data_dir
cfg.save_dataframe.output_file = 'fomc_tone_data_lm.parquet'
tone_data_lm = eKonf.instantiate(cfg)
tone_data_lm = eKonf.to_datetime(tone_data_lm, _columns='date')
tone_data_lm = tone_data_lm.set_index('date')
eKonf.save_data(tone_data_lm, 'fomc_tone_data_lm.parquet', data_dir)
INFO:ekorpkit.io.file:Processing [1] files from ['fomc_sents.parquet']
INFO:ekorpkit.io.file:Loading 1 dataframes from ['../data/fomc/fomc_sents.parquet']
INFO:ekorpkit.io.file:Loading data from ../data/fomc/fomc_sents.parquet
INFO:ekorpkit.pipelines.pipe:Applying pipeline: OrderedDict([('predict', 'predict'), ('aggregate_scores', 'aggregate_scores'), ('replace', 'replace'), ('pivot', 'pivot'), ('save_dataframe', 'save_dataframe')])
INFO:ekorpkit.base:Applying pipe: functools.partial(<function predict at 0x7f0abf86c820>)
INFO:ekorpkit.preprocessors.tokenizer:instantiating ekorpkit.preprocessors.stopwords.Stopwords...
INFO:ekorpkit.base:Calling load_candidates
INFO:ekorpkit.io.file:Processing [1] files from ['/workspace/projects/ekorpkit/ekorpkit/resources/lexicons/LM.parquet']
INFO:ekorpkit.io.file:Loading 1 dataframes from ['/workspace/projects/ekorpkit/ekorpkit/resources/lexicons/LM.parquet']
INFO:ekorpkit.io.file:Loading data from /workspace/projects/ekorpkit/ekorpkit/resources/lexicons/LM.parquet
INFO:ekorpkit.models.ngram.ngram:loaded 58142 candidates
INFO:ekorpkit.models.sentiment.lbsa:Predicting sentiments of the column [text] using predict_sentence
INFO:ekorpkit.base:Using batcher with minibatch size: 1000
INFO:ekorpkit.utils.batch.batcher: backend: joblib minibatch_size: 1000 procs: 100 input_split: False merge_output: True len(data): 653468 len(args): 5
Predicting [text]: 100%|██████████| 654/654 [03:06<00:00, 3.51it/s]
INFO:ekorpkit.models.sentiment.lbsa: >> elapsed time to predict: 0:03:07.279688
INFO:ekorpkit.pipelines.pipe:Saving data to: {'file': None, 'filename': 'fomc_sent_sentiments.parquet', 'base_dir': '../data/fomc', 'filetype': '', 'columns': None, 'suffix': None, 'filepath': '../data/fomc/fomc_sent_sentiments.parquet'}
INFO:ekorpkit.io.file:Saving dataframe to ../data/fomc/fomc_sent_sentiments.parquet
INFO:ekorpkit.base:Applying pipe: functools.partial(<function aggregate_scores at 0x7f0abf86c8b0>)
INFO:ekorpkit.base:instantiating ekorpkit.models.sentiment.base.BaseSentimentAnalyser...
INFO:ekorpkit.pipelines.pipe:filename not specified
INFO:ekorpkit.base:Applying pipe: functools.partial(<function general_function at 0x7f0abf86cc10>)
INFO:ekorpkit.pipelines.pipe:processing column: content_type
INFO:ekorpkit.pipelines.pipe: >> elapsed time to replace: 0:00:00.003644
INFO:ekorpkit.base:Applying pipe: functools.partial(<function pivot at 0x7f0abf86c1f0>)
INFO:ekorpkit.base:Applying pipe: functools.partial(<function save_dataframe at 0x7f0abf7043a0>)
INFO:ekorpkit.io.file:Saving dataframe to ../data/fomc/fomc_sentiment_data.parquet
polarity_mean_beigebook | polarity_mean_meeting_script | polarity_mean_minutes | polarity_mean_press_conf | polarity_mean_speech | polarity_mean_statement | polarity_mean_testimony | polarity_diffusion_beigebook | polarity_diffusion_meeting_script | polarity_diffusion_minutes | ... | num_tokens_sum_speech | num_tokens_sum_statement | num_tokens_sum_testimony | num_tokens_mean_beigebook | num_tokens_mean_meeting_script | num_tokens_mean_minutes | num_tokens_mean_press_conf | num_tokens_mean_speech | num_tokens_mean_statement | num_tokens_mean_testimony | |
---|---|---|---|---|---|---|---|---|---|---|---|---|---|---|---|---|---|---|---|---|---|
recent_meeting | |||||||||||||||||||||
1990-02-07 | NaN | -0.087583 | NaN | NaN | NaN | NaN | NaN | NaN | -0.095663 | NaN | ... | NaN | NaN | NaN | NaN | 30.213010 | NaN | NaN | NaN | NaN | NaN |
1990-03-27 | NaN | -0.171992 | NaN | NaN | NaN | NaN | NaN | NaN | -0.179702 | NaN | ... | NaN | NaN | NaN | NaN | 29.846369 | NaN | NaN | NaN | NaN | NaN |
1990-05-15 | NaN | -0.116052 | NaN | NaN | NaN | NaN | NaN | NaN | -0.125461 | NaN | ... | NaN | NaN | NaN | NaN | 29.749077 | NaN | NaN | NaN | NaN | NaN |
1990-07-03 | NaN | -0.114829 | NaN | NaN | NaN | NaN | NaN | NaN | -0.117794 | NaN | ... | NaN | NaN | NaN | NaN | 29.667920 | NaN | NaN | NaN | NaN | NaN |
1990-08-21 | NaN | -0.209552 | NaN | NaN | NaN | NaN | NaN | NaN | -0.219403 | NaN | ... | NaN | NaN | NaN | NaN | 31.032836 | NaN | NaN | NaN | NaN | NaN |
... | ... | ... | ... | ... | ... | ... | ... | ... | ... | ... | ... | ... | ... | ... | ... | ... | ... | ... | ... | ... | ... |
2021-06-16 | 0.011743 | NaN | 0.041638 | -0.017544 | -0.031786 | 0.435897 | 0.235955 | 0.012195 | NaN | 0.031142 | ... | 6894.0 | 384.0 | 2522.0 | 21.413279 | NaN | 30.615917 | 27.100000 | 29.088608 | 29.538462 | 28.337079 |
2021-07-28 | -0.120547 | NaN | -0.043969 | 0.021318 | -0.042941 | 0.461538 | NaN | -0.134921 | NaN | -0.069079 | ... | 13170.0 | 399.0 | NaN | 20.980159 | NaN | 32.888158 | 27.261628 | 29.072848 | 30.692308 | NaN |
2021-09-22 | -0.074328 | NaN | -0.079199 | -0.087292 | -0.133837 | 0.476190 | 0.031746 | -0.075712 | NaN | -0.112403 | ... | 31138.0 | 419.0 | 1026.0 | 22.957808 | NaN | 31.348837 | 25.809384 | 29.431002 | 29.928571 | 24.428571 |
2021-11-03 | -0.046022 | NaN | -0.064255 | -0.089881 | -0.030345 | 0.215686 | -0.120000 | -0.048109 | NaN | -0.080851 | ... | 26096.0 | 538.0 | 556.0 | 22.539497 | NaN | 31.880851 | 27.720238 | 31.980392 | 31.647059 | 27.800000 |
2021-12-15 | NaN | NaN | -0.043929 | -0.075441 | -0.356613 | 0.166667 | NaN | NaN | NaN | -0.064286 | ... | 3694.0 | 489.0 | NaN | NaN | NaN | 30.521429 | 37.587413 | 29.317460 | 27.166667 | NaN |
286 rows × 35 columns
cfg = eKonf.compose('pipeline')
cfg._pipeline_ = ['aggregate_scores', 'replace', 'pivot', 'save_dataframe']
cfg.num_workers = 100
cfg.data.data_file = "fomc_sent_sentiments.parquet"
cfg.data.data_dir = data_dir
cfg.aggregate_scores.groupby = ['content_type', 'date']
cfg.replace.apply_to = 'content_type'
cfg.replace.rcParams.to_replace = {'fomc_': ''}
cfg.replace.rcParams.regex = True
cfg.pivot.index = 'date'
cfg.pivot.columns = 'content_type'
cfg.pivot.values = ['polarity_mean', 'polarity_diffusion', 'num_examples', 'num_tokens_sum', 'num_tokens_mean']
cfg.save_dataframe.output_dir = data_dir
cfg.save_dataframe.output_file = 'fomc_tone_data_lm.parquet'
tone_data_lm = eKonf.instantiate(cfg)
tone_data_lm = eKonf.to_datetime(tone_data_lm, _columns='date')
tone_data_lm = tone_data_lm.set_index('date')
eKonf.save_data(tone_data_lm, 'fomc_tone_data_lm.parquet', data_dir)
Predict sentiments with the finbert#
ds_cfg = eKonf.compose('dataset')
ds_cfg.name = 'financial_phrasebank'
ds_cfg.path.cache.uri = 'https://github.com/entelecheia/ekorpkit-book/raw/main/assets/data/financial_phrasebank.zip'
ds_cfg.data_dir = ds_cfg.path.cached_path
ds_cfg.verbose = False
overrides=[
'+model/transformer=classification',
'+model/transformer/pretrained=finbert',
]
model_cfg = eKonf.compose('model/transformer=classification', overrides)
model_cfg.name = 'fomc_finbert'
model_cfg.dataset = ds_cfg
model_cfg.verbose = False
model_cfg.config.num_train_epochs = 2
model_cfg.config.max_seq_length = 256
model_cfg.config.train_batch_size = 32
model_cfg.config.eval_batch_size = 32
model_cfg._method_ = ['eval']
# model_cfg._method_ = ['train', 'eval']
finbert_model = eKonf.instantiate(model_cfg)
Accuracy: 0.8960176991150443
Precison: 0.8957698961367982
Recall: 0.8960176991150443
F1 Score: 0.8958088377661515
Model Report:
___________________________________________________
precision recall f1-score support
negative 0.81 0.77 0.79 61
neutral 0.96 0.96 0.96 277
positive 0.79 0.81 0.80 114
accuracy 0.90 452
macro avg 0.85 0.85 0.85 452
weighted avg 0.90 0.90 0.90 452
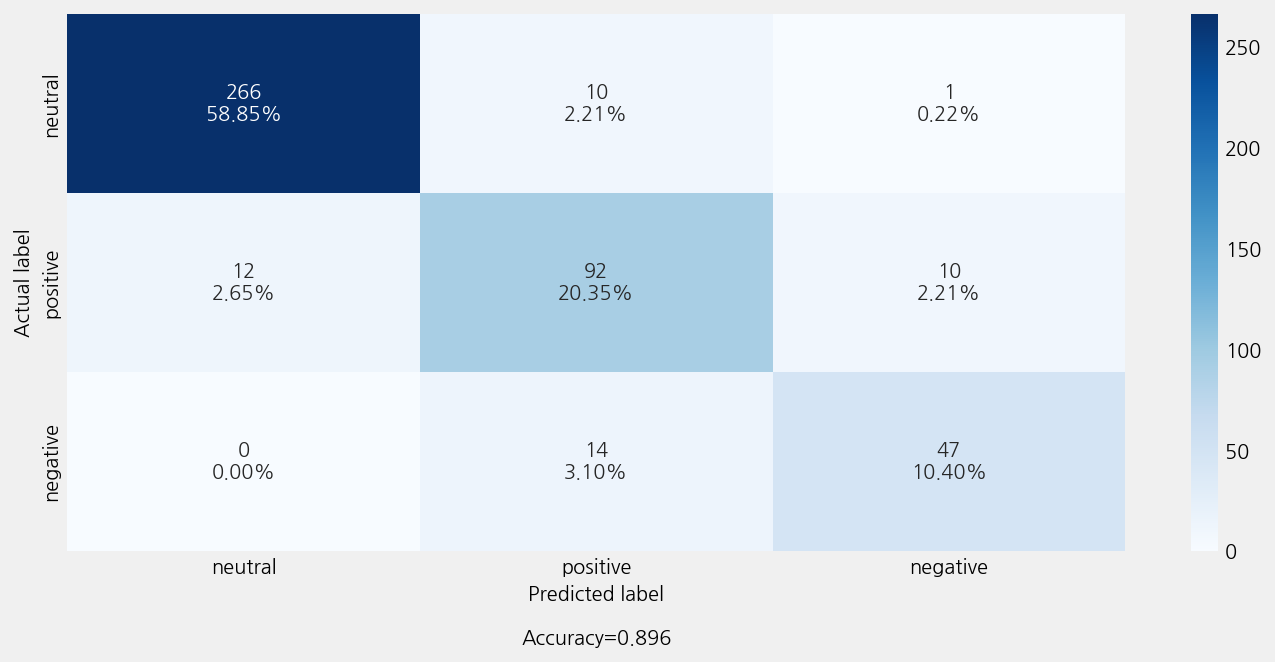
model_cfg._method_ = []
cfg = eKonf.compose(config_group='pipeline')
cfg.name = 'fomc_sent_sentiments'
cfg.data_dir = data_dir
cfg.data_file = "fomc_sents.parquet"
cfg._pipeline_ = ['predict']
cfg.predict.model = model_cfg
cfg.predict.output_dir = data_dir
cfg.predict.output_file = f'{cfg.name}_finbert.parquet'
fomc_sent_sentiments_finbert = eKonf.instantiate(cfg)
fomc_sent_sentiments_finbert.head()
id | text | split | timestamp | content_type | date | speaker | title | decision | rate | ... | recent_rate | next_meeting | next_decision | next_rate | text_num_words | section_id | sent_id | pred_labels | raw_preds | pred_probs | |
---|---|---|---|---|---|---|---|---|---|---|---|---|---|---|---|---|---|---|---|---|---|
0 | 0 | The Secretary reported that advices of the ele... | train | 1993-02-03 | fomc_minutes | 1993-02-03 | Alan Greenspan | FOMC Meeting Minutes | 0.0 | 3.0 | ... | 3.0 | 1993-02-18 | 0.0 | 3.0 | 47 | 29 | 0 | neutral | [2.2915966510772705, -0.9586986899375916, -2.4... | 0.955035 |
1 | 0 | By unanimous vote, the Committee elected the f... | train | 1993-02-03 | fomc_minutes | 1993-02-03 | Alan Greenspan | FOMC Meeting Minutes | 0.0 | 3.0 | ... | 3.0 | 1993-02-18 | 0.0 | 3.0 | 73 | 35 | 0 | neutral | [1.692587971687317, -0.2049560397863388, -2.53... | 0.858684 |
2 | 0 | By unanimous vote, William J. McDonough, Marga... | train | 1993-02-03 | fomc_minutes | 1993-02-03 | Alan Greenspan | FOMC Meeting Minutes | 0.0 | 3.0 | ... | 3.0 | 1993-02-18 | 0.0 | 3.0 | 74 | 37 | 0 | neutral | [1.8892569541931152, -0.3972317576408386, -2.6... | 0.898655 |
3 | 0 | On January 15, 1993, the continuing rules, reg... | train | 1993-02-03 | fomc_minutes | 1993-02-03 | Alan Greenspan | FOMC Meeting Minutes | 0.0 | 3.0 | ... | 3.0 | 1993-02-18 | 0.0 | 3.0 | 59 | 39 | 0 | neutral | [2.335063934326172, -1.0927255153656006, -2.45... | 0.960805 |
4 | 0 | Members were asked to indicate if they wished ... | train | 1993-02-03 | fomc_minutes | 1993-02-03 | Alan Greenspan | FOMC Meeting Minutes | 0.0 | 3.0 | ... | 3.0 | 1993-02-18 | 0.0 | 3.0 | 25 | 39 | 1 | neutral | [2.3842966556549072, -1.327033519744873, -2.36... | 0.967973 |
5 rows × 22 columns
fomc_sent_sentiments_finbert = eKonf.load_data('fomc_sent_sentiments_finbert.parquet', data_dir)
fomc_sent_sentiments_finbert
id | text | split | timestamp | content_type | date | speaker | title | decision | rate | ... | recent_rate | next_meeting | next_decision | next_rate | text_num_words | section_id | sent_id | pred_labels | raw_preds | pred_probs | |
---|---|---|---|---|---|---|---|---|---|---|---|---|---|---|---|---|---|---|---|---|---|
0 | 0 | The Secretary reported that advices of the ele... | train | 1993-02-03 | fomc_minutes | 1993-02-03 | Alan Greenspan | FOMC Meeting Minutes | 0.0 | 3.00 | ... | 3.00 | 1993-02-18 | 0.0 | 3.00 | 47 | 29 | 0 | neutral | [2.2915966510772705, -0.9586986899375916, -2.4... | 0.955035 |
1 | 0 | By unanimous vote, the Committee elected the f... | train | 1993-02-03 | fomc_minutes | 1993-02-03 | Alan Greenspan | FOMC Meeting Minutes | 0.0 | 3.00 | ... | 3.00 | 1993-02-18 | 0.0 | 3.00 | 73 | 35 | 0 | neutral | [1.692587971687317, -0.2049560397863388, -2.53... | 0.858684 |
2 | 0 | By unanimous vote, William J. McDonough, Marga... | train | 1993-02-03 | fomc_minutes | 1993-02-03 | Alan Greenspan | FOMC Meeting Minutes | 0.0 | 3.00 | ... | 3.00 | 1993-02-18 | 0.0 | 3.00 | 74 | 37 | 0 | neutral | [1.8892569541931152, -0.3972317576408386, -2.6... | 0.898655 |
3 | 0 | On January 15, 1993, the continuing rules, reg... | train | 1993-02-03 | fomc_minutes | 1993-02-03 | Alan Greenspan | FOMC Meeting Minutes | 0.0 | 3.00 | ... | 3.00 | 1993-02-18 | 0.0 | 3.00 | 59 | 39 | 0 | neutral | [2.335063934326172, -1.0927255153656006, -2.45... | 0.960805 |
4 | 0 | Members were asked to indicate if they wished ... | train | 1993-02-03 | fomc_minutes | 1993-02-03 | Alan Greenspan | FOMC Meeting Minutes | 0.0 | 3.00 | ... | 3.00 | 1993-02-18 | 0.0 | 3.00 | 25 | 39 | 1 | neutral | [2.3842966556549072, -1.327033519744873, -2.36... | 0.967973 |
... | ... | ... | ... | ... | ... | ... | ... | ... | ... | ... | ... | ... | ... | ... | ... | ... | ... | ... | ... | ... | ... |
653463 | 2854 | It will not have the word “somewhat” on line 3. | train | 2014-12-17 | fomc_meeting_script | 2014-12-17 | MR. LUECKE | FOMC Meeting Transcript | 0.0 | 0.25 | ... | 0.25 | 2015-01-28 | 0.0 | 0.25 | 10 | 287 | 2 | neutral | [2.3733553886413574, -1.3893874883651733, -2.2... | 0.967620 |
653464 | 2854 | Chair Yellen Yes Vice Chairman Dudley ... | train | 2014-12-17 | fomc_meeting_script | 2014-12-17 | MR. LUECKE | FOMC Meeting Transcript | 0.0 | 0.25 | ... | 0.25 | 2015-01-28 | 0.0 | 0.25 | 31 | 287 | 4 | neutral | [2.224238872528076, -0.893900990486145, -2.421... | 0.948905 |
653465 | 2854 | And let me confirm that the next meeting will ... | train | 2014-12-17 | fomc_meeting_script | 2014-12-17 | CHAIR YELLEN | FOMC Meeting Transcript | 0.0 | 0.25 | ... | 0.25 | 2015-01-28 | 0.0 | 0.25 | 19 | 288 | 3 | neutral | [2.349479913711548, -1.391977071762085, -2.298... | 0.967770 |
653466 | 2854 | I believe box lunches are now available for pe... | train | 2014-12-17 | fomc_meeting_script | 2014-12-17 | CHAIR YELLEN | FOMC Meeting Transcript | 0.0 | 0.25 | ... | 0.25 | 2015-01-28 | 0.0 | 0.25 | 33 | 288 | 4 | neutral | [2.311434030532837, -1.1415460109710693, -2.37... | 0.960746 |
653467 | 2854 | I will do my best, and I will consider at the ... | train | 2014-12-17 | fomc_meeting_script | 2014-12-17 | CHAIR YELLEN | FOMC Meeting Transcript | 0.0 | 0.25 | ... | 0.25 | 2015-01-28 | 0.0 | 0.25 | 18 | 288 | 5 | neutral | [2.3932976722717285, -1.2607381343841553, -2.4... | 0.967106 |
653468 rows × 22 columns
cfg = eKonf.compose('pipeline')
cfg._pipeline_ = ['aggregate_scores', 'replace', 'pivot', 'save_dataframe']
cfg.num_workers = 100
cfg.data.data_file = "fomc_sent_sentiments_finbert.parquet"
cfg.data.data_dir = data_dir
cfg.aggregate_scores.groupby = ['content_type', 'date']
cfg.aggregate_scores._method_ = 'classification'
cfg.replace.apply_to = 'content_type'
cfg.replace.rcParams.to_replace = {'fomc_': ''}
cfg.replace.rcParams.regex = True
cfg.pivot.index = 'date'
cfg.pivot.columns = 'content_type'
cfg.pivot.values = ['polarity_mean', 'polarity_diffusion', 'num_examples']
cfg.save_dataframe.output_dir = data_dir
cfg.save_dataframe.output_file = 'fomc_sentiment_finbert_next.parquet'
tone_data_finbert = eKonf.instantiate(cfg)
tone_data_finbert = eKonf.to_datetime(tone_data_finbert, _columns='date')
tone_data_finbert = tone_data_finbert.set_index('date')
eKonf.save_data(tone_data_finbert, 'fomc_tone_data_finbert.parquet', data_dir)
tone_data_finbert = eKonf.load_data('fomc_tone_data_finbert.parquet', data_dir)
cols = [
'polarity_mean_minutes', 'polarity_mean_press_conf', 'polarity_mean_speech', 'polarity_mean_statement',
'polarity_diffusion_minutes', 'polarity_diffusion_press_conf', 'polarity_diffusion_speech', 'polarity_diffusion_statement',
]
tone_data_finbert = tone_data_finbert[cols].copy()
tone_data_finbert.columns = tone_data_finbert.columns.str.replace('polarity', 'finbert')
tone_data_finbert
finbert_mean_minutes | finbert_mean_press_conf | finbert_mean_speech | finbert_mean_statement | finbert_diffusion_minutes | finbert_diffusion_press_conf | finbert_diffusion_speech | finbert_diffusion_statement | |
---|---|---|---|---|---|---|---|---|
date | ||||||||
1990-02-07 | NaN | NaN | NaN | NaN | NaN | NaN | NaN | NaN |
1990-03-27 | NaN | NaN | NaN | NaN | NaN | NaN | NaN | NaN |
1990-05-15 | NaN | NaN | NaN | NaN | NaN | NaN | NaN | NaN |
1990-07-03 | NaN | NaN | NaN | NaN | NaN | NaN | NaN | NaN |
1990-08-21 | NaN | NaN | NaN | NaN | NaN | NaN | NaN | NaN |
... | ... | ... | ... | ... | ... | ... | ... | ... |
2021-11-30 | NaN | NaN | 0.182338 | NaN | NaN | NaN | 0.239583 | NaN |
2021-12-01 | NaN | NaN | NaN | NaN | NaN | NaN | NaN | NaN |
2021-12-02 | NaN | NaN | 0.262141 | NaN | NaN | NaN | 0.338889 | NaN |
2021-12-15 | 0.509806 | 0.280516 | NaN | 0.412947 | 0.675 | 0.377622 | NaN | 0.555556 |
2021-12-17 | NaN | NaN | 0.408242 | NaN | NaN | NaN | 0.547619 | NaN |
1876 rows × 8 columns
Predict sentiments with the T5#
ds_cfg = eKonf.compose('dataset')
ds_cfg.name = 'financial_phrasebank'
ds_cfg.path.cache.uri = 'https://github.com/entelecheia/ekorpkit-book/raw/main/assets/data/financial_phrasebank.zip'
ds_cfg.data_dir = ds_cfg.path.cached_path
ds_cfg.verbose = False
overrides=[
'+model/transformer=t5_classification_with_simple',
'+model/transformer/pretrained=t5-base',
]
model_cfg = eKonf.compose('model/transformer=t5_classification_with_simple', overrides)
model_cfg.name = 'fomc_t5'
model_cfg.dataset = ds_cfg
model_cfg.verbose = False
model_cfg.config.num_train_epochs = 2
model_cfg.config.max_seq_length = 256
model_cfg.config.train_batch_size = 8
model_cfg.config.eval_batch_size = 8
model_cfg._method_ = ['train', 'eval']
# model_cfg._method_ = ['eval']
t5_model = eKonf.instantiate(model_cfg)
/opt/conda/lib/python3.8/site-packages/transformers/models/t5/tokenization_t5.py:164: FutureWarning: This tokenizer was incorrectly instantiated with a model max length of 512 which will be corrected in Transformers v5.
For now, this behavior is kept to avoid breaking backwards compatibility when padding/encoding with `truncation is True`.
- Be aware that you SHOULD NOT rely on t5-base automatically truncating your input to 512 when padding/encoding.
- If you want to encode/pad to sequences longer than 512 you can either instantiate this tokenizer with `model_max_length` or pass `max_length` when encoding/padding.
- To avoid this warning, please instantiate this tokenizer with `model_max_length` set to your preferred value.
warnings.warn(
/opt/conda/lib/python3.8/site-packages/transformers/tokenization_utils_base.py:3557: FutureWarning:
`prepare_seq2seq_batch` is deprecated and will be removed in version 5 of HuggingFace Transformers. Use the regular
`__call__` method to prepare your inputs and the tokenizer under the `as_target_tokenizer` context manager to prepare
your targets.
Here is a short example:
model_inputs = tokenizer(src_texts, ...)
with tokenizer.as_target_tokenizer():
labels = tokenizer(tgt_texts, ...)
model_inputs["labels"] = labels["input_ids"]
See the documentation of your specific tokenizer for more details on the specific arguments to the tokenizer of choice.
For a more complete example, see the implementation of `prepare_seq2seq_batch`.
warnings.warn(formatted_warning, FutureWarning)
wandb: Currently logged in as: entelecheia. Use `wandb login --relogin` to force relogin
wandb version 0.12.20 is available! To upgrade, please run:
$ pip install wandb --upgrade
Tracking run with wandb version 0.12.19
Run data is saved locally in
/workspace/projects/ekorpkit-book/outputs/fomc_t5/t5-base/wandb/run-20220701_020155-3fyvrvgf
/opt/conda/lib/python3.8/site-packages/transformers/tokenization_utils_base.py:3557: FutureWarning:
`prepare_seq2seq_batch` is deprecated and will be removed in version 5 of HuggingFace Transformers. Use the regular
`__call__` method to prepare your inputs and the tokenizer under the `as_target_tokenizer` context manager to prepare
your targets.
Here is a short example:
model_inputs = tokenizer(src_texts, ...)
with tokenizer.as_target_tokenizer():
labels = tokenizer(tgt_texts, ...)
model_inputs["labels"] = labels["input_ids"]
See the documentation of your specific tokenizer for more details on the specific arguments to the tokenizer of choice.
For a more complete example, see the implementation of `prepare_seq2seq_batch`.
warnings.warn(formatted_warning, FutureWarning)
{'eval_loss': 0.06258307859733529}
Accuracy: 0.9491150442477876
Precison: 0.9495910765330818
Recall: 0.9491150442477876
F1 Score: 0.9474806981643329
Model Report:
___________________________________________________
precision recall f1-score support
negative 0.96 0.75 0.84 61
neutral 0.97 1.00 0.98 277
positive 0.90 0.94 0.92 114
accuracy 0.95 452
macro avg 0.94 0.90 0.91 452
weighted avg 0.95 0.95 0.95 452
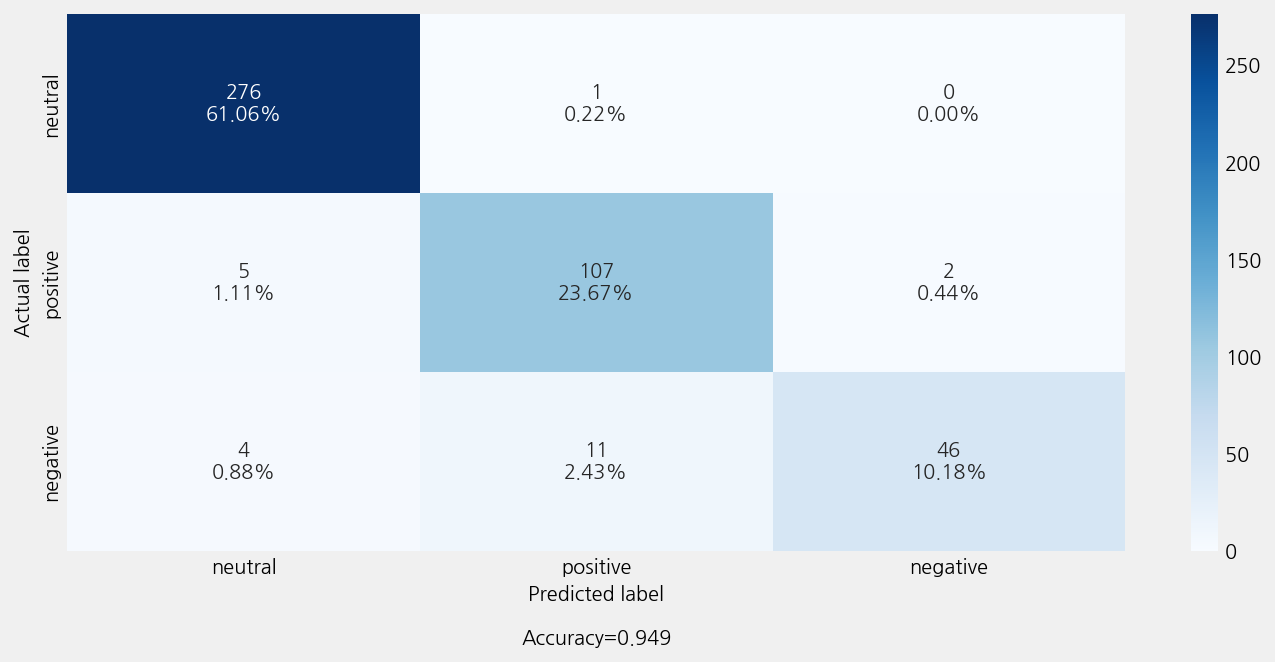
model_cfg._method_ = []
cfg = eKonf.compose(config_group='pipeline')
cfg.name = 'fomc_sent_sentiments'
cfg.data_dir = data_dir
cfg.data_file = "fomc_sents.parquet"
cfg._pipeline_ = ['predict']
cfg.predict.model = model_cfg
cfg.predict.output_dir = data_dir
cfg.predict.output_file = f'{cfg.name}_t5.parquet'
fomc_sent_sentiments_t5 = eKonf.instantiate(cfg)
fomc_sent_sentiments_t5.head()
/opt/conda/lib/python3.8/site-packages/transformers/tokenization_utils_base.py:3557: FutureWarning:
`prepare_seq2seq_batch` is deprecated and will be removed in version 5 of HuggingFace Transformers. Use the regular
`__call__` method to prepare your inputs and the tokenizer under the `as_target_tokenizer` context manager to prepare
your targets.
Here is a short example:
model_inputs = tokenizer(src_texts, ...)
with tokenizer.as_target_tokenizer():
labels = tokenizer(tgt_texts, ...)
model_inputs["labels"] = labels["input_ids"]
See the documentation of your specific tokenizer for more details on the specific arguments to the tokenizer of choice.
For a more complete example, see the implementation of `prepare_seq2seq_batch`.
warnings.warn(formatted_warning, FutureWarning)
id | text | split | timestamp | content_type | date | speaker | title | decision | rate | ... | recent_decision | recent_rate | next_meeting | next_decision | next_rate | text_num_words | section_id | sent_id | prefix | pred_labels | |
---|---|---|---|---|---|---|---|---|---|---|---|---|---|---|---|---|---|---|---|---|---|
0 | 0 | The Secretary reported that advices of the ele... | train | 1993-02-03 | fomc_minutes | 1993-02-03 | Alan Greenspan | FOMC Meeting Minutes | 0.0 | 3.0 | ... | 0.0 | 3.0 | 1993-02-18 | 0.0 | 3.0 | 47 | 29 | 0 | classification | neutral |
1 | 0 | By unanimous vote, the Committee elected the f... | train | 1993-02-03 | fomc_minutes | 1993-02-03 | Alan Greenspan | FOMC Meeting Minutes | 0.0 | 3.0 | ... | 0.0 | 3.0 | 1993-02-18 | 0.0 | 3.0 | 73 | 35 | 0 | classification | neutral |
2 | 0 | By unanimous vote, William J. McDonough, Marga... | train | 1993-02-03 | fomc_minutes | 1993-02-03 | Alan Greenspan | FOMC Meeting Minutes | 0.0 | 3.0 | ... | 0.0 | 3.0 | 1993-02-18 | 0.0 | 3.0 | 74 | 37 | 0 | classification | neutral |
3 | 0 | On January 15, 1993, the continuing rules, reg... | train | 1993-02-03 | fomc_minutes | 1993-02-03 | Alan Greenspan | FOMC Meeting Minutes | 0.0 | 3.0 | ... | 0.0 | 3.0 | 1993-02-18 | 0.0 | 3.0 | 59 | 39 | 0 | classification | neutral |
4 | 0 | Members were asked to indicate if they wished ... | train | 1993-02-03 | fomc_minutes | 1993-02-03 | Alan Greenspan | FOMC Meeting Minutes | 0.0 | 3.0 | ... | 0.0 | 3.0 | 1993-02-18 | 0.0 | 3.0 | 25 | 39 | 1 | classification | neutral |
5 rows × 21 columns
cfg = eKonf.compose('pipeline')
cfg._pipeline_ = ['aggregate_scores', 'replace', 'pivot', 'save_dataframe']
cfg.num_workers = 100
cfg.data.data_file = "fomc_sent_sentiments_t5.parquet"
cfg.data.data_dir = data_dir
cfg.aggregate_scores.groupby = ['content_type', 'date']
cfg.aggregate_scores._method_ = 'classification_t5'
cfg.replace.apply_to = 'content_type'
cfg.replace.rcParams.to_replace = {'fomc_': ''}
cfg.replace.rcParams.regex = True
cfg.pivot.index = 'date'
cfg.pivot.columns = 'content_type'
cfg.pivot.values = ['polarity_diffusion', 'num_examples']
cfg.save_dataframe.output_dir = data_dir
cfg.save_dataframe.output_file = 'fomc_sentiment_t5_next.parquet'
tone_data_t5 = eKonf.instantiate(cfg)
tone_data_t5 = eKonf.to_datetime(tone_data_t5, _columns='date')
tone_data_t5 = tone_data_t5.set_index('date')
eKonf.save_data(tone_data_t5, 'fomc_tone_data_t5.parquet', data_dir)
tone_data_t5 = eKonf.load_data('fomc_tone_data_t5.parquet', data_dir)
cols = [
'polarity_diffusion_minutes', 'polarity_diffusion_press_conf', 'polarity_diffusion_speech', 'polarity_diffusion_statement',
]
tone_data_t5 = tone_data_t5[cols].copy()
tone_data_t5.columns = tone_data_t5.columns.str.replace('polarity', 't5')
tone_data_t5
t5_diffusion_minutes | t5_diffusion_press_conf | t5_diffusion_speech | t5_diffusion_statement | |
---|---|---|---|---|
date | ||||
1990-02-07 | NaN | NaN | NaN | NaN |
1990-03-27 | NaN | NaN | NaN | NaN |
1990-05-15 | NaN | NaN | NaN | NaN |
1990-07-03 | NaN | NaN | NaN | NaN |
1990-08-21 | NaN | NaN | NaN | NaN |
... | ... | ... | ... | ... |
2021-11-30 | NaN | NaN | 0.239583 | NaN |
2021-12-01 | NaN | NaN | NaN | NaN |
2021-12-02 | NaN | NaN | 0.250000 | NaN |
2021-12-15 | 0.403571 | 0.216783 | NaN | 0.444444 |
2021-12-17 | NaN | NaN | 0.174603 | NaN |
1876 rows × 4 columns