GPT-4#
Overview#
GPT-4 Overview:#
GPT-4 is OpenAI’s most sophisticated system, providing safer and more practical responses. It boasts enhanced general knowledge, problem-solving skills, and creativity.
Improved Performance:#
GPT-4 demonstrates superior reasoning abilities compared to ChatGPT, scoring higher on tests like the Uniform Bar Exam and Biology Olympiad.
Safety & Alignment:#
OpenAI has invested 6 months in refining GPT-4’s safety and alignment, incorporating human feedback, expert consultations, and lessons from real-world usage of previous models.
GPT-4-assisted Safety Research:#
GPT-4’s advanced capabilities have accelerated safety work, with the model assisting in creating training data and iterating on classifiers.
Collaborations:#
GPT-4 has been used by organizations like Duolingo, Be My Eyes, Stripe, Morgan Stanley, Khan Academy, and the Government of Iceland for various innovative applications.
Research, Infrastructure & Limitations:#
GPT-4 is a milestone in scaling up deep learning, trained on Microsoft Azure AI supercomputers. OpenAI acknowledges its limitations and aims to address them while promoting transparency and AI literacy.
Availability:#
GPT-4 is accessible through ChatGPT Plus and an API, allowing developers to build applications and services using the model.
GPT-4 Technical Report#
Summary#
GPT-4: large multimodal model processing image and text inputs
Goal: improve natural language understanding and generation
Performance: excels in various exams, outperforms previous models and state-of-the-art systems
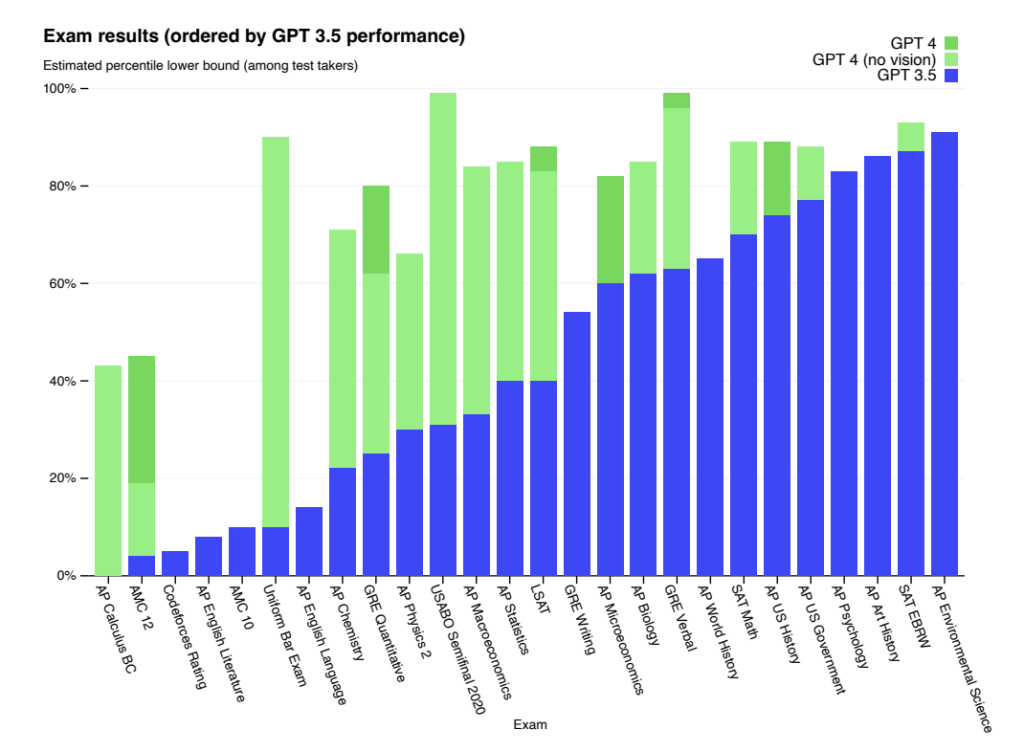
Fig. 136 GPT performance on academic and professional exams#
MMLU benchmark: surpasses English state-of-the-art in 24 of 26 languages
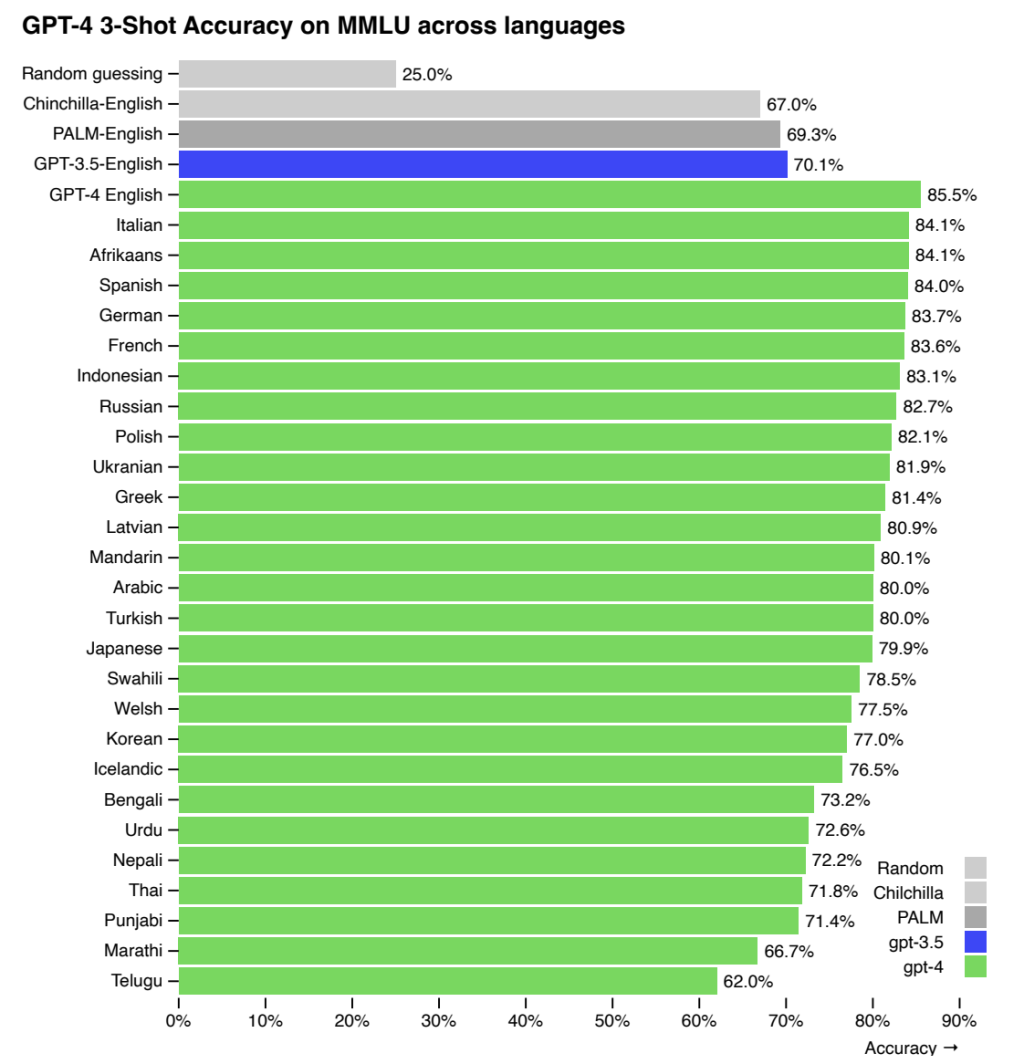
Fig. 137 Performance of GPT-4 in a variety of languages compared to prior models in English on MMLU.#
Infrastructure challenge: predictable deep learning optimization across scales
Limitations: not fully reliable, limited context window, doesn’t learn from experience
Safety challenges: bias, disinformation, over-reliance, privacy, cybersecurity, proliferation
Interventions: adversarial testing, domain expert input, model-assisted safety pipeline
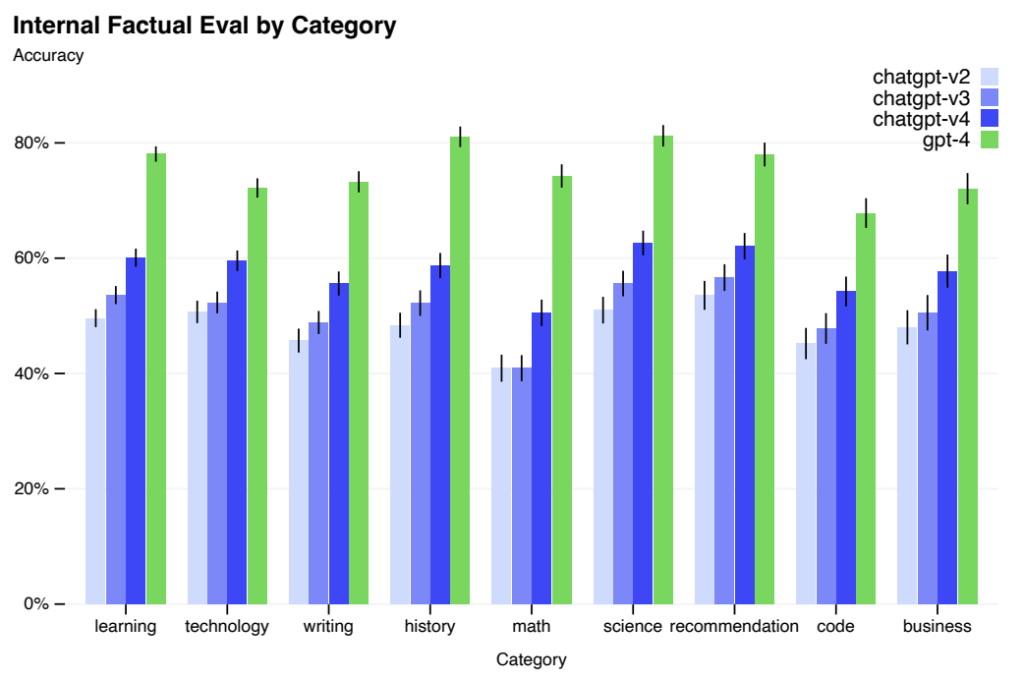
Fig. 138 Performance of GPT-4 on nine internal adversarially-designed factuality evaluations.#
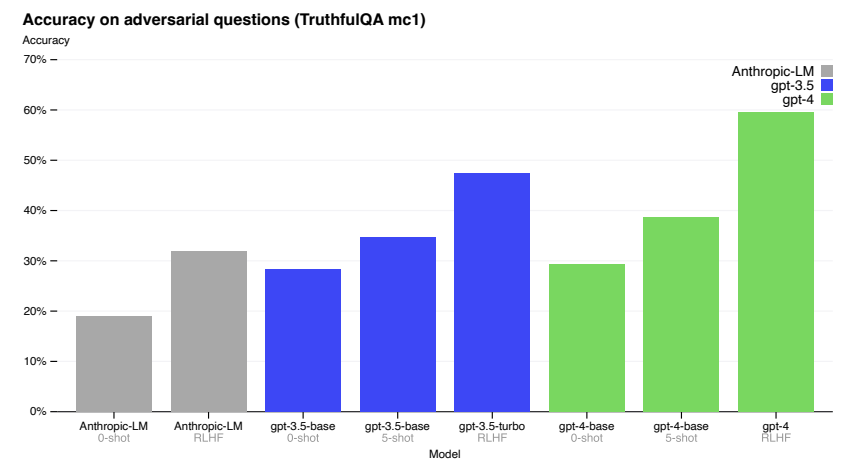
Fig. 139 Performance of GPT-4 on TruthfulQA.#
Predictable scaling: accurate predictions on loss and capabilities
Conclusion#
Implemented safety measures: reduced harmful content generation, but vulnerabilities remain
Critical need: extremely high reliability in interventions and use policies and monitoring
Continue learning from deployment: update models for safety and alignment
Key steps for language model developers:
Adopt layers of mitigations throughout the model system
Build evaluations, mitigations, and deployment with real-world usage in mind
Ensure safety assessments cover emergent risks
Plan for capability jumps “in the wild”
Encourage research into:
Economic impacts of AI and increased automation
Broader public participation in decisions about model behavior
Evaluations for risky emergent behaviors
Interpretability, explainability, calibration, and AI literacy
Need for more research in AI literacy, economic/social resilience, and anticipatory governance
Importance of further development in evaluation tools and technical improvements in model safety
GPT-4 System Card#
LLMs deployed in various domains: browsing, voice assistants, coding assistance tools
GPT-4: latest LLM in GPT family
Safety challenges: convincing but false text, illicit advice, dual-use capabilities, risky emergent behaviors
OpenAI safety processes: measurements, model-level changes, product/system-level interventions, external expert engagement
Mitigations alter GPT-4 behavior, prevent some misuses, but remain limited and brittle
Need for anticipatory planning and governance